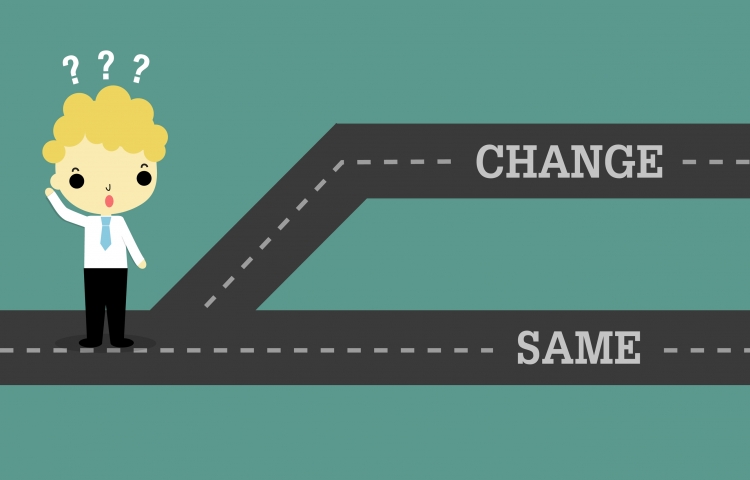
Rethinking Quantifying Behavior Change: A Case for New Uses, Measures, and Analysis
By: John Torous and Sherry Pagoto
We often hear about new use cases for technologies like smartphones apps and wearable to quantify behavior with the goal of inducing behavioral changes. Track steps to walk more, log food to eat healthier, record medications to boost adherence. But evidence has shown that this simple premise often fails - just recording and presenting people with their own data rarely induces long-term behavioral changes. What if the problem was actually not how we quantify behavior with technology but rather how we use, measure, and analyze behavior change data? The right collaborations between behavior change, data science, and design experts will help address today’s challenges and offer ready-to-implement solutions.
While the saying that it takes 10,000 hours to develop new expertise may be either an over- or underestimate for any particular person, it is certainly the case that behavior change takes time. While some people who decided to stop smoking never picked up another cigarette again, for most it takes between five to ten failed attempts before quitting for good. This is very different from the world of clinical studies where we often only look at a single attempt at behavior change – and on a very short timeframe. Thus, understanding the long-term impact of technology on behavior change requires long-term measurement. Technologies like wearable sensors or smartphone apps have the potential for such long-term monitoring – but people often stop using wearables or stop tracking with an app within months. To realize the full potential of technology for behavior change we need to design these devices and programs to be used for years, not days, and consider engagement on a longer and larger scale. Taking into account the correct time frame to see the real impact of behavior change can help set the context and needs for technology use. Integrating the right human factors design, data collection, and behavioral science theory for such long-term success is possible when experts from these different disciplines collaborate.
Another issue in quantifying behavior change with technology is that it is surprisingly difficult to reproduce or validate results from studies. A core of science is reproducibility and showing the same behavioral intervention works over and over again – but this is often challenging because technology keeps evolving so rapidly. In order to compare results across studies, populations, and use cases, we need common standards and units to quantify behavior change. Currently measurements such as steps are frequently used in many digital health studies. But what exactly is a step? In the physical activity scientific literature, accelerometry remains the gold standard for measurement as it is accurate and well validated. In contrast, measured steps can vary wildly between different wearable devices and apps and there is still no consensus regarding the clinical significance of the commonly targeted 10,000 steps per day for people. Exercise science has long quantified physical activity as minutes spent in varying intensities of physical activity--a metric that has strong associations with morbidity and mortality (unlike steps). In an era of precision medicine and quantified behavior change – we need to use meaningful, precision measurements wherever possible instead of imprecise ones that lack predictive ability. Matching the right behavioral change theory with valid measurements to create engaging and impactful technology solutions is possible with the right expertise at the table.
But even with the right uses and measurements for behavior change, one further challenge is the analysis of behavior change data itself. In this era of digital monitoring with sensor, smartphones, social media etc., - there is a glut of data. While more data offers many benefits, it also increases risk of finding spurious or random associations that are statistically significant but clinically insignificant. The massive amount of behavior change data available in this digital age means that today it is critical that behavior change experts like psychologists and psychiatrists work closely with data scientists to gauge both the statistical and clinical significance of the questions being asked in the data and the results. Such partnerships are useful not only to avoid false or meaningless findings, but also can help define better uses and more efficient statistical methods that can take behavior change data further and uncover new insights. Likewise, data scientists need to work closely with behavior change experts to ensure they are applying large behavior change data sets towards meaningful and relevant problems. A simple question of “how will this impact patients or users and improve their life?” can often lead to an informative team discussion and reframing of what behavior change techniques and data the technology solution should incorporate.
Quantifying behavior change in the digital era offers tremendous promise to learn about both personal and population-level behaviors that can lead to better lives and healthier communities. But quantifying behavior change in the digital era also offers new challenges in the form of finding the right uses, measures, and analysis to uncover meaningful results.
John Torous, MD is co-director of the digital psychiatry program at Beth Israel Deaconess Medical Center, a Harvard Medical School affiliated teaching hospital, where he also serves as a staff psychiatrist and clinical informatics fellow.
Sherry Pagoto, PhD is a Professor in the Department of Allied Health Sciences at the University of Connecticut and Director of the UConn Center for mHealth and Social Media. She tweets at @DrSherryPagoto.